The Proposed OAFormer Approach
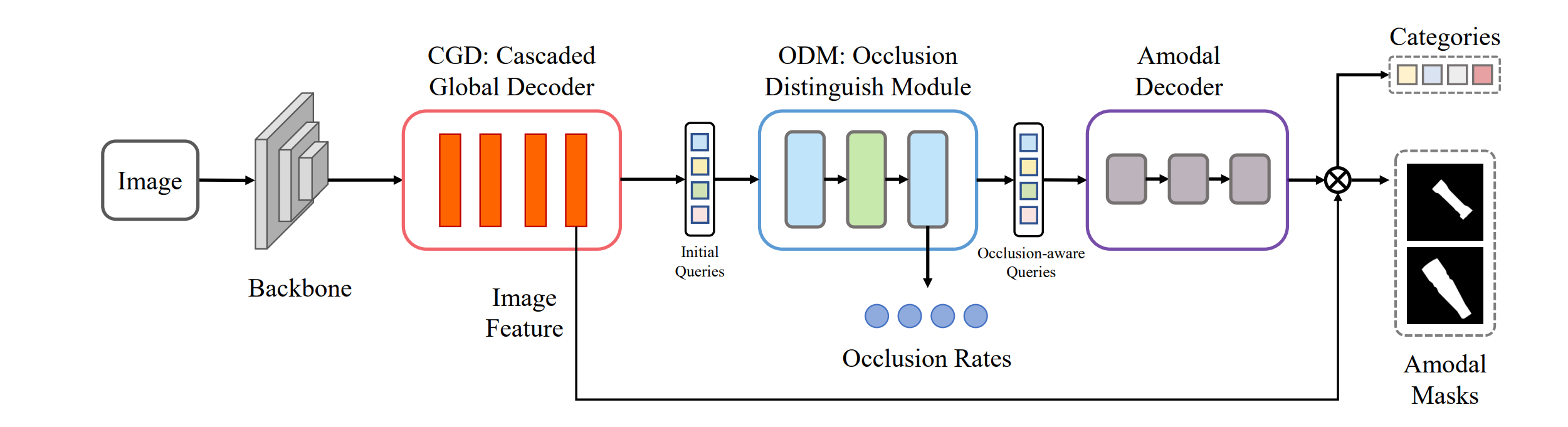
Overview of the proposed OAFormer. OAFormer takes an image as the input. After extracting the features by the Encoder and the Cascaded Global Decoder, the Occlusion Distinguish Module predicts the occlusion rates of each target objects and embeds occlusion information into the attention masks. Finally, the Amodal Decoder takes the occlusion-aware attention masks and queries as input, and outputs the predicted amodal masks.