The Proposed AURA Approach
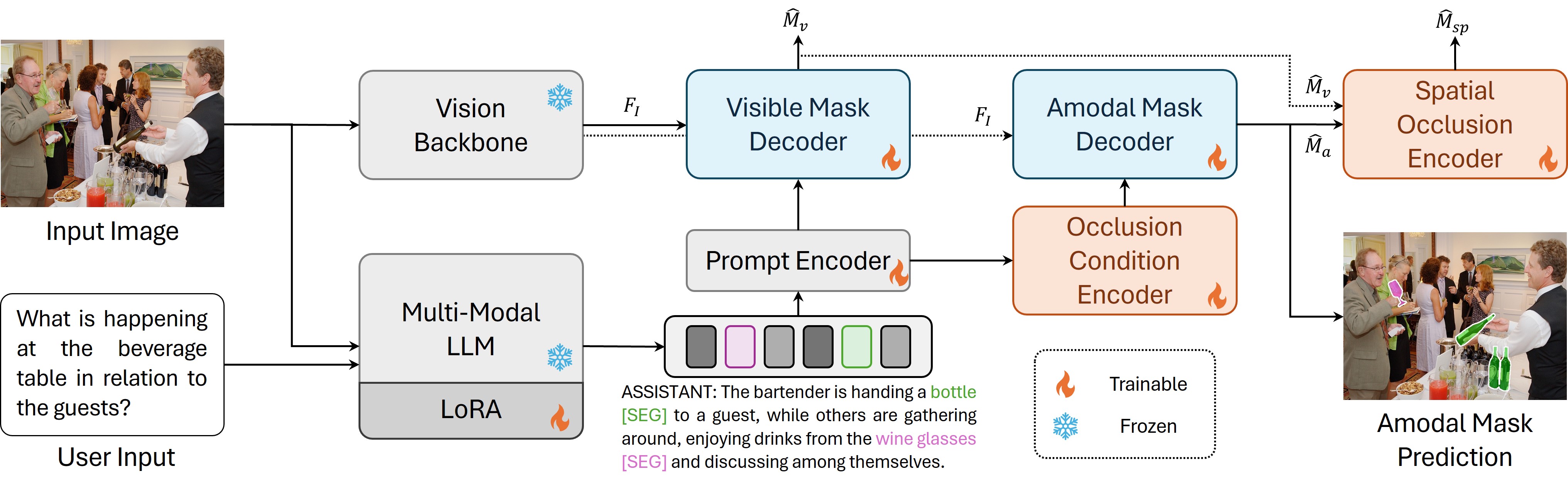
Overall architecture of the proposed AURA. (a) Given an input image and the input question from the user, the Vision Backbone extracts the visual features of the input image, and the Multi-Modal LLM equipped with LoRA is utilized for understanding the input image and textual questions simultaneously and responding with textual answers including the [SEG] tokens indicating the segmentation masks. (b) For each [SEG], the Prompt Encoder takes its embedding of the Multi-Modal LLM and outputs the refined embeddings corresponding to the [SEG]. (c) Finally, the Visible Mask Decoder predicts the visible mask using each [SEG]'s refined embeddings. The Amodal Decoder predicts the amodal mask using the occlusion-aware embedding predicted by the Occlusion Condition Encoder. A Spatial Occlusion Encoder is designed to constrain the spatial occlusion information of the predicted visible and amodal segmentation masks to be accurate.